FAIR Annotation of Bioassay Data – SciBite
Learn how SciBite unlock the value of bioassay data through semantic enrichment of metadata to create FAIR annotation.
- Unified annotation from ontologies across an area of business
- Standardized metadata for any application
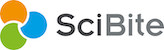
Overview
One of the most valuable assets for any organisation is its data. This is especially true of pre-clinical data; created and collected after carrying out a multitude of biological experimental assays. Databases dedicated to managing bioassay data contain an amazing wealth of Research & Development knowledge, providing a rich resource for mining with both scientific and operational questions. Many hurdles exist when it comes to extracting knowledge from these resources, including the fact that many companies deploy data management systems that are geared towards entering rather than mining data and/or replacing such systems over time which results in silos of legacy data in a variety of formats aligned to a multitude of different standards. When implementing a change in data management strategy, it should not be limited to legacy data. Based on the FAIR principles, and using semantic enrichment, SciBite unlock the value of bioassay data by coupling retrospective analysis of existing data with controlled prospective data entry [1].
Process
Bioassay data management systems are often based on relational databases. While this affords some structure to data, the associated front-end applications tend to capture data as free text fields to avoid burdening or restricting users. In addition, even for more defined entries, the meaning of a particular field or its contents may be ambiguous, imprecise or contain multiple different data types, such as Gene, Target and Species. Similarly, inconsistent use of synonyms during data entry makes it difficult to collate data for a disease or target of interest. For example, a search of a typical bioassay database for the Alzheimer’s related gene, PSEN1, would miss references to synonyms such as Presenilin-1, AD3 and PSNL1. The normalisation of literature and alignment of text to ontologies and industry standards is vital to truly make data FAIR (see Figure 1).
Figure 1. Normalising entities captured in bioassay titles. Extraction of Cell Line, Drug, Species and Target entities within the unstructured titles of a selection of assays. The resulting semantic index enables connections to be made between bioassays
When applying standard, well established ontologies and controlled vocabularies to bioassay data, the source of ontologies is a key consideration as the use of a proprietary ontology results in reliance on a specific vendor. By using public standards, such as BAO (BioAssay Ontology), ChEMBL (chemical entities), CLO (Cell Ontology) and EFO (Experimental Factor Ontology), the resulting enriched data is open and interoperable from system to system, which is fundamental to FAIR.
Implementing a change in data management strategy should not be limited to legacy data but should also enable data that is prospectively captured to be aligned to the same ontological standards so as to ensure seamless integration of historic and future data. With this in mind, this use case outlines a workflow that uses ontology management combined with semantic enrichment to unlock the value of bioassay data (see Figure 2) which contains the following steps:
- Selection and management of relevant public ontologies using the ontology editing and management tool, CENtree.
- Historic data normalisation and alignment to the selected ontologies. For example, all synonyms and variations of the term “mouse” (mice, Mus musculus), will be aligned to the same term [Mus musculus] using a named entity recognition and extraction engine, TERMite.
- Control over the data input to ensure that the entry term is aligned to the correct class in the relevant ontology using a form manegemt tool, SciBite Forms. This has autocomplete functionality to enable an organisation to achieve semantic enrichment of their data in real-time and at the point of capture. Instead of being presented with restrictive and lengthy drop-down menus, users can enter text into semantically aware fields and have relevant terms suggested to them as they type.
This workflow ensures that bioassay data and metadata contains 1) identifiers that can be queried easily and intelligently (Findable), so that appropriate users can gain computational access (Accessible), 2) can be integrated with other data sources (Interoperable) and 3) is richly described and understandable, following community standards (Reusable).
Figure 2. Bioassay registration workflow. Workflow shows how SciBite technology can be used to enable the FAIRification of bioassay data, both retrospectively and prospectively.
Outcomes
The approach above enables semantic enrichment of bioassay data. Enrichment not only makes it simpler to interrogate bioassay data, ensuring that all relevant data is found regardless of which synonym was used as the search term, it also facilitates more complex ontology-based questions to be asked of the data that would otherwise not be possible. For example, it may be of interest to ask the following questions of your assay data, something that is made possible by the workflow described.
- Which targets have we studied that are associated with inflammatory disorders?
- Which diseases have we studied for both a target of interest and other targets in the same class and what were the outcomes?
- Which assays have utilised a rodent cell line?
- Which protein kinases have we run screens for (and how many screens have we done for each one)?
- Which experimental techniques are growing across the organisation and would benefit from a core facility?
Furthermore, once data is normalised and aligned to ontologies, the task of enriching such data with alternative data sources, be it external or internal data sources, becomes a trivial one; meaning additional evidence from sources can be integrated to automate the data analysis steps.
This use case combines retrospective and prospective data management, which has been deployed by a number of SciBite’s customers. It brings intelligent scientific search to any bioassay platform, to make bioassay data computationally accessible for automated analysis, ensuring realisation of its full value.
References and Resources
- SciBite https://www.scibite.com
At a Glance
Team
- 1 FTE knowledge engineer/ontologist(s)
- 1 FTE subject matter expert(s)
Timeline
- 2-3 months
- Shorter timeframe if several experts are involved
Deliverables & benefits
- Unified annotation from ontologies across an area of business
- Standardized metadata for use by any application
- Education of the business in the implementation of FAIR data
Authors
Top Tips
- Bioassay data are consistently and unambiguously tagged with key metadata
- Enables the wealth of information in bioassay databases to be unlocked and exploited
- FAIR annotation of bioassay FAIR data and metadata will include appropriate identifiers