The Role of Curated Data in Accelerating the Identification of Putative Drug Targets for Cancer Immunotherapies – Elucidata
Elucidata tackles challenges in finding and reusing data through its curation app and custom curation of relevant data.
-
Reduced turnaround time; unlocked previously inaccessible datasets
-
Transformed target identification timeline in cancer immunotherapy
Overview
Basic data preprocessing steps include- searching for relevant data, identifying suitable datasets, and processing them through a consistent pipeline. These must be followed to validate results, identify an active biomolecular entity, or for any other downstream application.
“The most relevant datasets are never identified, let alone used.”
Elucidata transforms biological discovery by providing high-quality bulk RNA-seq and single-cell data, among other data types. In this case study, we discuss a specific use case where a leading genomics-based drug discovery company in California, USA, wanted to accelerate the identification of putative targets across immunological diseases and cancer. Their primary approach was utilizing publicly available data to complement and validate their research, but they encountered difficulties locating and reusing data. In order to fully capitalize on the available research data and facilitate its direct use in their downstream pipelines, they formed a partnership with Elucidata. The collaboration aimed to identify and curate relevant public data, and customizing it to suit specific research needs.
Process
Deriving value from biological data is an immensely challenging task due to the heterogeneous nature of the data, the wide variety of formats and repositories in which it is stored, and the semi-structured way it is shared (with information scattered across tables and text). Here, we discuss in detail how Elucidata teamed up with the therapeutics company to address the challenge of finding and standardizing pertinent data to identify putative targets through a tailored curation process.
Biological data curation refers to systematically organizing and integrating data acquired from diverse sources. This process encompasses annotation, data standardization, and metadata harmonization using a uniform ontology. Curation ensures the data is Findable, Accessible, Interoperable, and Reusable. Though the broader definition of curation is widely accepted, it is not a ‘one-size-fits-all’ solution. Depending on the research question, the fields that need to be curated will change. In this case, the pharma company was looking for datasets with specific information around fields such as cancer staging, gender, etc., to aid in their quest to find putative targets across immunological diseases and cancer. They needed these tailor-made fields apart from the usual standard fields such as disease, organism, tissue, etc. Custom curation of these fields eased data selection and enhanced data usability by following a standard system for different entities and allowed the data to be directly used downstream. Let’s take a look at the process involved.
There are 4 major steps in the journey to customized, deeply curated biomedical data:
- Finding relevant data
- Establishing guidelines
- Custom curation of datasets
- Delivering the data in a readily consumable form

The end-to-end process
Let’s take a deep dive into each of these parts.
Finding Relevant Data:
The research team had identified active small molecules and wanted to generate and test drug formulations based on those. They wanted different data types – transcriptomics, snp array, single-cell RNA seq, proteomics – related to normal and cancerous samples from the liver, kidney, and skin. The first step was to set up a data audit.
Based on a deep understanding of the biological context of the customer’s data requirements, a dashboard with metadata from relevant datasets mined from various repositories and publications was generated. This was possible in a short span of 1-2 weeks because Elucidata’s data platform, Polly, hosts ~40,000 highly curated datasets from different sources that are curated with the help of domain trained Natural Language Processing (NLP) models (We will cover the curation process in detail later in this document). Relevant data that was not on the platform was also mined using various pipelines and brought to the platform to generate a comprehensive data dashboard. The dashboard showed the distribution of the organisms, data types, and sources involved. This helped the team understand the available data more granularly and shortlist the datasets most suitable to their research.
Establishing the Guidelines for the New Fields to Be Curated
For each new field to be curated, it is essential to prepare detailed guidelines. In collaboration with the research team, our project team developed a comprehensive framework to guide the curation process.
Special emphasis was laid on details such as:
- Datasets of interest (along with the number of datasets)
- Specific study or disease of interest
- New fields to be curated
- Preference for any specific ontologies for requested custom fields
- A brief idea of the consumption journey (optional)* *Here, the customer chose to divulge some details of the intended downstream processes. This information helped fine-tune the curation process.
Clear guidelines ensure that the curation process remains consistent, transparent, and reproducible. Consistent curation is vital for data reuse and helped the company use the data directly on their automated pipelines and for downstream analyses, thereby reducing their data processing time significantly.
Curating the Data
Once the guidelines were fixed, the next step was to check the source of the datasets. The process was more straightforward for datasets on our biomedical data platform, Polly, as these datasets were already auto-curated by NLP models. Datasets from external sources were ingested through standard ETL (extract, transform, load) pipelines. All the required metadata were indexed properly at this stage to aid further curation. At Elucidata, NLP models and experts work hand in hand to streamline the curation process. We use an in-house developed ‘Curation app’ and expert-assisted manual curation/validation to complete the curation process and QC accurately.
The Curation app is a data annotation tool for labeling multiple data types for metadata fields. It has an integrated active learning system that uses machine learning (ML) to provide annotation suggestions to increase the speed of curation and create NLP models that can curate data by themselves. This app is powered by a domain-trained BERT model, Polly BERT, which has been shown to have human-level accuracy in several named-entity recognition (NER) tasks. Read more here. Using the curation app, different types of curation can be performed at the dataset level (in free text and tabular format) and the sample level (in tabular format). At Elucidata, we use expert-assisted manual curation process where two curators independently approve the curation suggestions brought out by the curation app (double-blinded curation). This is followed by a QC process where an expert reviews the results. This ensures maximum accuracy without compromising on speed.
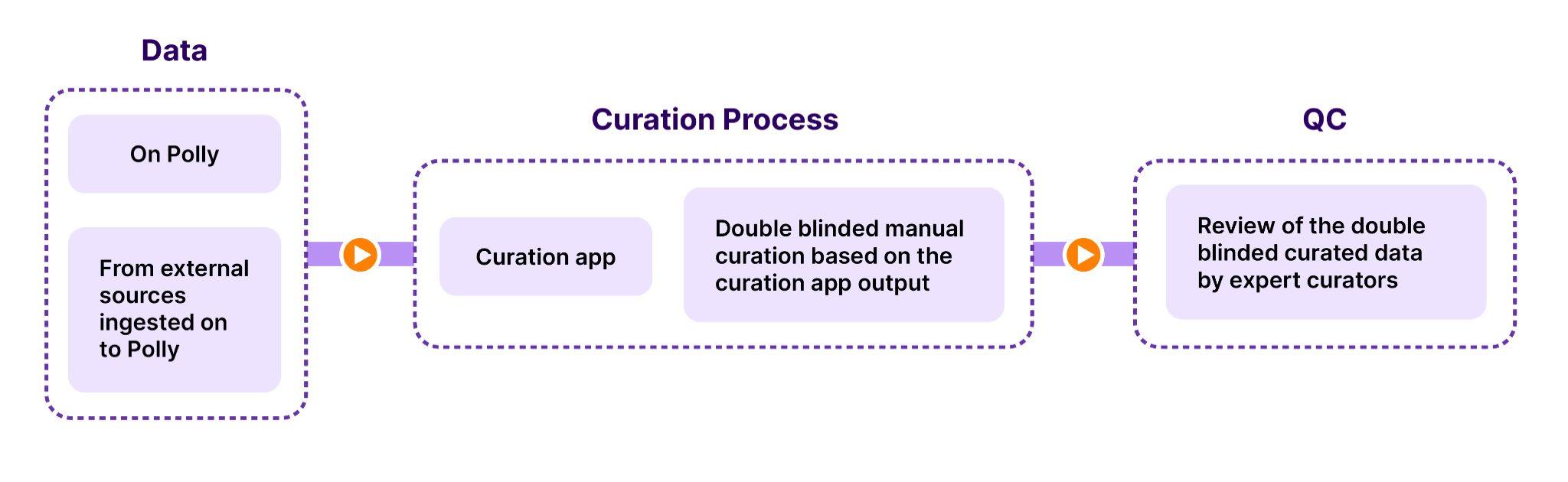
The curation workflow
Data Delivery
Elucidata’s data warehouse, OmixAtlas, can store biomedical data in a structured format ready for downstream machine learning and analytical applications. Once the datasets are curated, they are hosted on OmixAtlas, where the research team can access and analyze them. As per their requirement, the curated data was also shared in .json or .csv format.
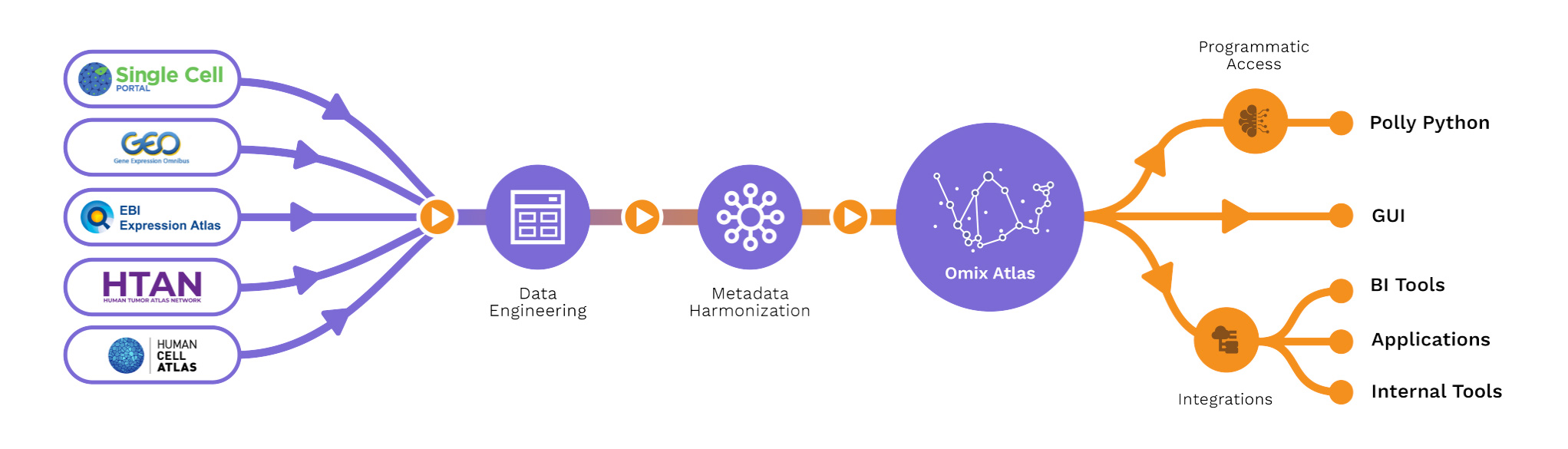
The data processing pipeline
Outcomes
This effective partnership of process, technology & people allows Elucidata to scale up the curation of complex biological data accurately and consistently.
Some of the remarkable achievements are listed below:
- Turnaround time (TAT) reduction in dataset search and curation. TAT for up to 10 datasets (up to 4 new fields): 2-4 days; if scaled to 100 datasets, the TAT is just 4-8 days. This was a critical consideration as the oncology company wanted to use all the relevant datasets available on the topic.
- Created a curated multiple-cancer immune atlas using transcriptomics data from GEO and fast-tracked the identification of 1 target in just 6 months, compared to the usual 2-3 years.
- Enabled data-oriented tools and workflows
- Secured datasets that would otherwise be disregarded due to findability and usability issues
- Identified new patterns related to drug resistance, stage-specific differences, and gene-survival association from the data provided.
References and Resources
- Wilkinson MD et al 2019 Evaluating FAIR maturity through a scalable, automated, community-governed framework. Sci. Data 6, 174. doi.org/10.1038/s41597-019-0184-5
- https://docs.polly.elucidata.io/OmixAtlas/OmixAtlas.html
- https://fairtoolkit.pistoiaalliance.org/fair-guiding-principles/
- Mukund Chaudhry Chaudhry, Arman Kazmi, Shashank Jatav, Akhilesh Verma, Vishal Samal, Kristopher Paul, and Ashutosh Modi. 2022. Reducing Inference Time of Biomedical NER Tasks using Multi-Task Learning. In Proceedings of the 19th International Conference on Natural Language Processing (ICON), pages 116–122, New Delhi, India. Association for Computational Linguistics.
At a Glance
Team:
Customer success manager, Technical SME, Delivery SME, Expert Curators
Timeline:
6 months
Deliverables:
Curated data resources
- 7500+ samples across 2 sources and 5 data types
- 70 deeply curated, extremely relevant datasets with 13 curated fields, which could be directly ingested into their downstream pipelines.
Professional services
- Data adaptors in development
- Incorporated the above resources into broader ecosystem of the customer’s tools
Tooling
- Cell x Gene for scRNAseq
- Phantasus for bulk RNAseq
The cost saved (ROI): ~ 80 – 90% of the total estimated cost of curation without Polly
Below is the breakdown of the total estimated cost of curation without Polly ($761k).
Curation costs:
- Estimated curation costs of 25 minutes per sample
- 3,125 total hours estimated for 7,500 samples – Internal cost of ~$546k (at $175/h)
- Additional 1,000 hours for light curation of 23k samples – internal cost of ~$175,000
Data search costs
- ~19 hours per month typically used to audit data
- Estimated internal cost of ~$39.9k
Top Tips
- The initial discovery calls with the customer are pivotal in the project’s efficiency and pace. Understanding their needs helped us streamline the curation process to make data input to their infrastructure seamless.
- Curation is a dynamic need. The parameters for curation need to be designed based on the problem at hand.
-
- Curation depth is important for analysis with established interest areas
- Breadth (volume of datasets) is important for exploratory analyses