Sharing FAIR Data about HealthCare Partners – Bayer
Discover how Bayer builds a harmonised FAIR data asset based on Health Care Professional partners.
- A knowledge graph from federated data integration
- Enables reuse by different consumers
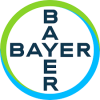
Overview
Along our whole R&D value chain we engage with Health Care Professionals (HCPs), as partners for research collaborations, as investigators in clinical trials or other medical studies, as key thought leaders in disease areas of interest, as direct contacts to our patients and prescribers of our products.
A wealth of data is collected but scattered across many systems within Bayer and found in data sources outside of Bayer. The data is not integrated and we cannot produce a 360-degree picture of individual HCPs since we cannot easily match identities and integrate the information.
We use the FAIR data principles as guidance to integrate data around HCPs in a data asset in order to make better informed decisions on research collaborations with HCPs.
Process
We defined and followed a data asset implementation cycle consisting of four steps:
1) Identify use cases, competency questions and decision points with a relevant business value
In order to build meaningful assets, we think it essential to start with a use case. This guides us to integrate the right data sources and provide them to data consumers in the required detail, format, quality and speed. Just think of an asset on healthcare professionals. It requires quite a different set of data if you want to answer questions around recruitment performance or the publication track record.
2) Focus on data and domain, define key data sources and subject matter experts
Next, we took a close look at the data sources and discussed content, structure, format and demands with the subject matter experts, i.e. application manager, data scientists, data consumers. The FAIRness of data was evaluated by a questionnaire which was adapted from the FAIR matrix (ref to go FAIR). In order to make data sources more Findable and Accessible, they are registered via the Bayer Corporate Linked Data (COLID) service.
3) Implement FAIR asset
To kick-start the implementation, we performed a FAIR datathon (similar to ref to method – BYOD workshop).
- This workshop format brings together a cross-functional team consisting of business representatives / subject matter experts, knowledge engineers, data architects, data scientists and application manager
- By “bringing your own data” participants figure out how FAIR their data is and learn in detail what the FAIR data principles mean.
- Together the team builds a data model around the knowledge domain relevant for the use case(s)/competency questions.
- We show which tools and services are available at Bayer to make data FAIR and do data curation.
- We show how, by using semantic technologies, the data can be integrated, and competency questions answered.
- Additional to the content created, this workshop format promotes a cross-functional and collaborative working mode.
The results of the workshop are then implemented in a sustainable and scalable platform and services for data ingestion, data integration and data provisioning – the data asset. The implementation of the assets includes the FAIRification process, especially focusing on Interoperability and Reusability. Data is curated, enriched with meta information, mapped to knowledge representations already existing and extended.
4) Usage platform and APIs
The cloud-native data asset platform is not yet another silo solution but integrates and builds on existing services developed by our internal IT teams. This encompasses the whole technical implementation, considering performance demands, e.g. ingestion of streaming data, huge complex data files, access management, persistence layers, data virtualization, semantic integration, and access layers for data consumers, primarily SPARQL, SQL or REST endpoints. The integrated data can then be consumed by a variety of applications, in our case a Tableau dashboard.
Outcomes
Bayer invests into research activities both initiated and conducted by external third parties r to advance medical and scientific knowledge and provide new value for patients in their treatments. For example, the outcomes of a sponsored study (SELECT-D, DOI: 10.1200/JCO.2018.78.8034) on rivaroxaban led to an update of ISTH guidelines (doi.org/10.1111/jth.14219). We wanted to increase the outcome of sponsored studies by collaborating with the most promising HCPs, sites and trials. In a first step we integrated publication information on HCPs in order to understand the publication performance and field of expertise.
Via our data asset implementation cycle we incorporated three external licensed data sources (PubMed, PharmaSpectra and Clarivate) and made the data around HCPs available in a dashboard. The dashboard is “just” one app providing data to consumers; the real value of the solution lies in the FAIRness of the data that we provided or are going to provide in the next increments.
- F: The sources are registered in our data market place, thus we created transparency on data sources we own
- A: We make the data accessible via flexible APIs to serve different requirements.
- I: We created an ontology to describe the HCP with attributes required by the use case. We re-used concepts of public ontologies (e.g. schema.org) and cross-referenced to existing concepts in other Bayer domain ontologies (e.g. Clinical Ontology).
- R: the HCP concepts described, and the data linked are available also for other use cases. We currently work on a machine-readable description of license information to bring more transparency on the data usage restrictions/rules.
We are currently doing an internal roadshow to show the results of the use case in order to collect more use cases / data sources around the HCP, as well as promoting our approach of the data asset implementation cycle.
References and Resources
- Clarivate: https://mjl.clarivate.com/home
- PharmaSpectra: https://www.pharmaspectra.com/solution/link
- PubMed: https://www.nlm.nih.gov/databases/download/pubmed_medline.html
At a Glance
Team
- product owner
- scrum master
- knowledge engineer
- data architect
- data scientist
- code developer
- subject matter expert
- UI designer
Timeline
- 3 months for first increment
Deliverables & benefits
- HealthCare professional knowledge graph
- FAIR data sources
- Data-centric architecture platform
- Federated data integration via virtualization
- Data is reusable for different consumers
Author
- Alexandra Grebe de Barron, Global Data Assets, Digital Transformation & IT, Bayer Pharmaceuticals (LinkedIn)
Top Tips
- Do early hands-on team work (e.g. FAIR datathon)
- Do fast prototyping
- Reach out to other initiatives and stakeholders